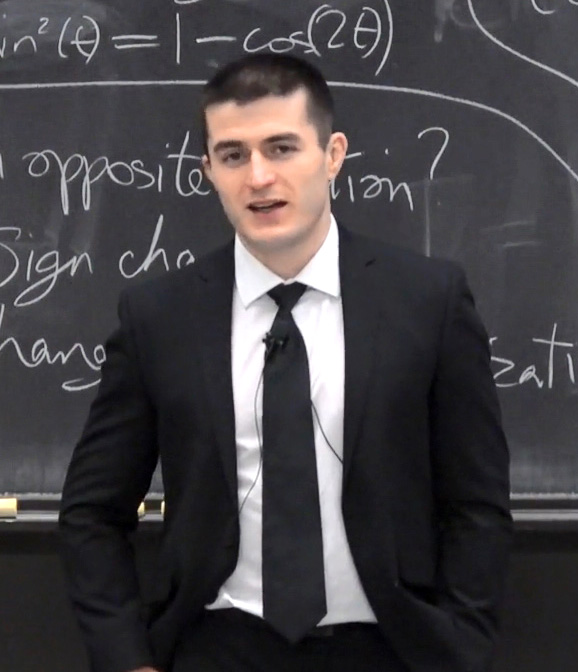
Lex Fridman
(pronounced: Freedman)
Research Scientist, MIT, 2015 - current (2023)
Laboratory for Information and Decision Systems (LIDS)
Research: Human-robot interaction and machine learning.
Hiring: I'm hiring
Teaching: deeplearning.mit.edu
Podcast: Lex Fridman Podcast
Sample Conversations:
Elon Musk,
Mark Zuckerberg,
Sam Harris,
Joe Rogan,
Vitalik Buterin,
Grimes,
Dan Carlin,
Roger Penrose,
Jordan Peterson,
Richard Dawkins,
Liv Boeree,
Leonard Susskind,
David Fravor,
Kanye West,
Donald Hoffman,
Rick Rubin,
etc.
Connect with me @lexfridman on Twitter, LinkedIn, Instagram, Facebook, YouTube, Medium.
Outside of research and teaching, I enjoy:
- playing guitar & piano
- practicing jiu jitsu & judo
Research & Publications (Google Scholar)
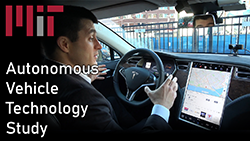
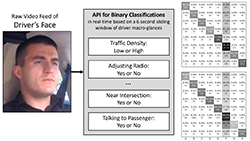
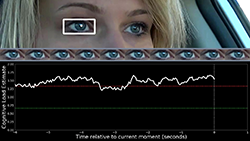
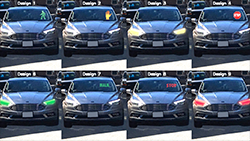
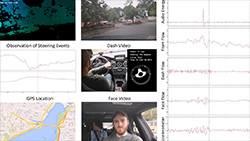
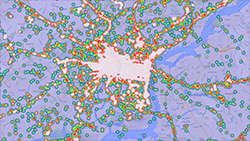
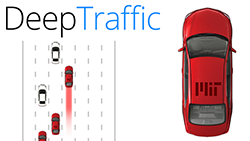
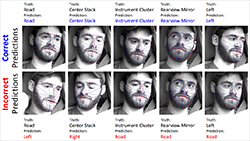
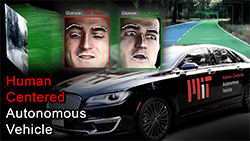
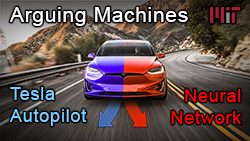